Introduction
As technology breakthroughs continue to push the boundaries of financial forecasting, the topic of “Can deep learning predict stock price?” is becoming more relevant. Predicting stock prices is a difficult and multifaceted task that is influenced by many variables, including investor sentiment and economic data. A branch of artificial intelligence called deep learning provides useful tools to help deal with this complexity. The dynamics of stock prices, traditional and contemporary prediction techniques, and the application of deep learning to financial market forecasting will all be covered in this blog article.
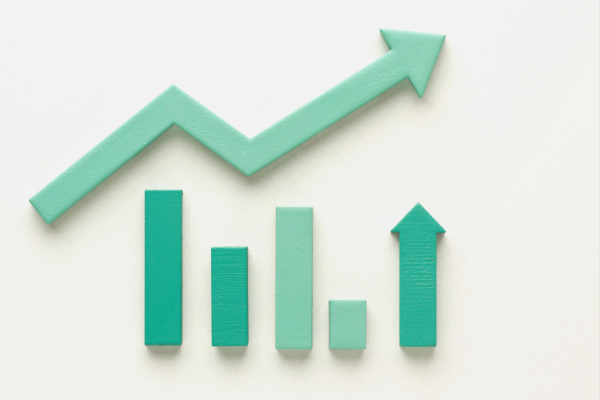
Table of Contents
Stock Prices and Market Dynamics
Many factors, such as firm performance, prevailing economic conditions, market mood, and geopolitical developments, influence stock prices. Market dynamics are intrinsically complex because of the way these factors interact, which makes prediction difficult. Given that these dynamics involve both macroeconomic and microeconomic elements, understanding them is essential for any prediction model.
Traditional Methods of Stock Price Prediction
Before the development of deep learning, statistical models, technical analysis, and fundamental analysis were the mainstays of stock price prediction. Technical analysis focuses on past price movements and trading volumes, while fundamental analysis looks at a company’s financial health and performance metrics. To find trends and patterns in stock prices, statistical models such as ARIMA (autoregressive integrated moving average) have also been used extensively.
Why is deep learning important for stock price prediction?
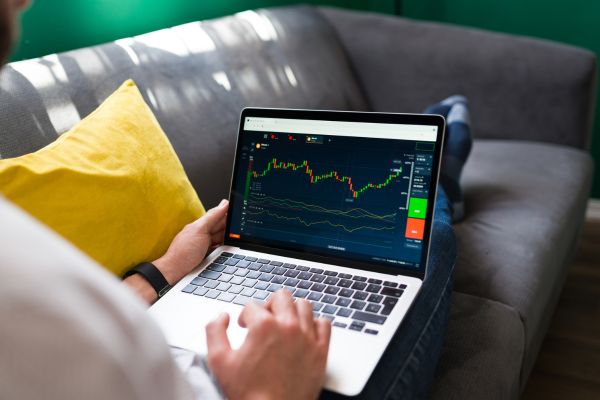
Because deep learning can handle large amounts of data and recognize complex patterns that standard methods overlook, it is crucial for stock price prediction. Deep learning models have the ability to automatically identify relevant features from raw data, unlike standard models that often require human feature engineering. With the noisy and multivariate nature of financial market data, this skill is very helpful.
Types of Deep Learning Models Used in Finance
In finance, different types of deep learning models are used, such as:
- Recurrent Neural Networks (RNN): These models are suitable for time-series forecasting, such as stock prices, as they are built for sequential data.
- An RNN type called Long Short-Term Memory Network (LSTM) is helpful in capturing patterns over long periods of time as it can learn long-term dependencies.
- Convolutional Neural Nets (CNN): Originally developed for image processing, CNNs can be used to find complex patterns in financial data.
- Generative Adversarial Networks (GAN): These artificial intelligence tools can produce synthetic data and enhance training datasets to improve model performance.
How Neural Networks Process Financial Data
To handle financial data, neural networks draw knowledge from past prices, trading volumes, and other relevant signals. Typically, data is normalized before being fed into the network, which minimizes prediction error by learning to modify its weights and biases. Deep learning models are effective tools for predicting stock prices because they can capture non-linear correlations and interactions between multiple factors.
Success Stories of Deep Learning in Stock Prediction
Several financial institutions and hedge funds have successfully applied deep learning for stock prediction. For example, J.P. Morgan uses machine learning algorithms, including deep learning, to enhance trading strategies and risk management. Similarly, Renaissance Technologies, a hedge fund known for its quantitative approach, uses sophisticated machine learning models to achieve higher returns.
Notable Errors and the Lessons They Teach Us
Deep learning has been used in finance, although not all of its applications have proven effective. The dangers associated with relying too heavily on automated models have been highlighted by the 2018 flash collapse, which was primarily blamed on algorithmic trading. The above-mentioned drawbacks highlight the importance of thorough testing, validation, and human supervision when using deep learning models.
Challenges and Limitations
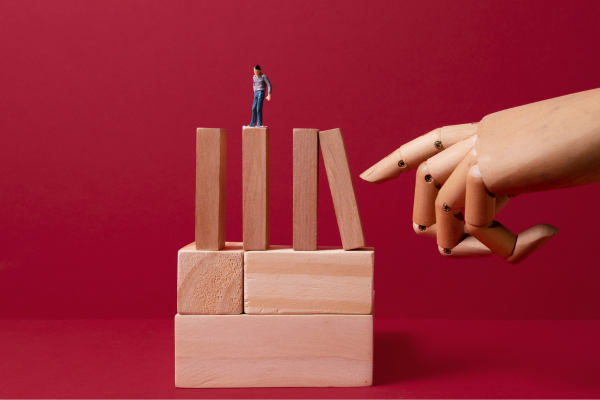
Challenges and Limitations
Financial data is inherently noisy and influenced by many factors, making it difficult to model accurately. Deep learning models require a very high volume of high-quality data, which can be a significant bottleneck in financial applications.
Overfitting and Other Common Pitfalls
When a model performs well on training data but performs poorly on unknown data, it is called overfitting, which is a prevalent problem in deep learning. Techniques such as regularization, cross-validation, and the use of simpler models can help mitigate it.
Ethical and practical considerations
Ethical implications of automated trading
Deep learning-powered automated trading presents serious ethical issues. One of these is the potential for market manipulation, in which algorithms can take advantage of inefficiencies in the market to gain an unfair advantage. Concerns have also been raised over job displacement when human traders are replaced by computerized technology. To guarantee the equitable and responsible use of these technologies, strong supervision and ethical requirements are necessary due to the dangers of unintended effects associated with reliance on sophisticated models.
Regulatory and compliance issues
The regulation of deep learning applications in finance is becoming more stringent. Regulatory agencies aim to maintain stability, equity, and openness in financial markets. Companies using deep learning models must comply with these restrictions. This includes complying with legislation protecting data privacy, guaranteeing algorithmic transparency, and preventing discriminatory behavior. Companies need to deal with this complex regulatory environment in order to properly use deep learning while maintaining compliance and trust.
Conclusion
Predicting stock prices may change even more thanks to cutting-edge technology such as blockchain and quantum computing. For example, quantum computing has the potential to improve the performance of deep learning models by solving complex optimization issues far more quickly than traditional computers.Can deep learning forecast stock price? The answer is complicated. Deep learning is a robust tool for stock price prediction, but its effectiveness depends on many aspects, such as model choice, data quality, and ethical issues. It is a useful part of a comprehensive financial plan rather than a magic bullet.
- You might be interested in reading these posts as well
- How do machine learning applications work?
- Does deep learning require feature engineering?
- Can deep learning applications generate images?
FAQs
Deep learning is a branch of artificial intelligence that processes data and creates patterns to make decisions by mimicking the functions of the human brain.
No, because financial markets are inherently unpredictable, deep learning algorithms can increase forecast accuracy but may not deliver accurate results.
In fact, there are risks associated with automated trading, including models overfitting, reliance on high-quality data, and ethical issues.
While traditional techniques rely on human feature engineering and more basic statistical models, deep learning is able to automatically recognize complex patterns from vast amounts of data.
The future looks bright, as improvements in algorithms and technology such as quantum computing should improve the predictive power of deep learning models.