Introduction
We embark on a journey to the realms where quantum computing and machine learning collide, diving deep into the complex network of opportunities and advancements. We explore the secrets and possibilities of these two domains while maintaining a laser-like focus on their symbiotic relationship.
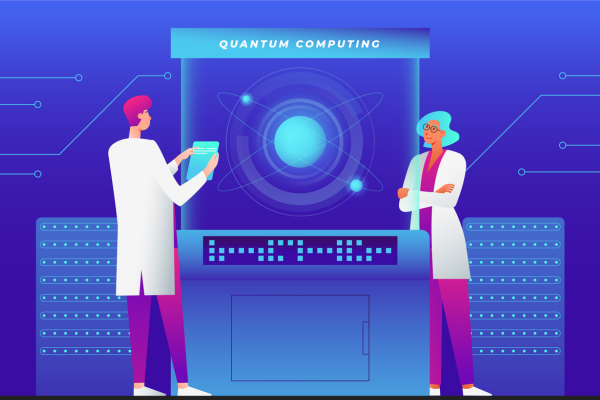
Table of Contents
The ability of machine learning and quantum computing to work together
The combination of quantum computing with machine learning is a shining example of innovation in a changing field of technology. There is immense potential for both these sectors to work together to solve difficult issues that were previously considered intractable. While quantum principles can enhance the capabilities of machine learning models, machine learning algorithms can take advantage of the inherent power of quantum computers, resulting in unprecedented breakthroughs in many fields.
Overview of Machine Learning Algorithms in Quantum Computing
The application of machine learning methods in the field of quantum computing is critical to this synergy. By bridging the gap between the classical and quantum computing paradigms, these algorithms allow the use of quantum phenomena to address a wide range of computational tasks. Machine learning algorithms open the door to revolutionary advances in quantum computing research, from quantum-enhanced optimization to the construction of quantum generator models.
How quantum computing is being revolutionized by machine learning techniques?
The marriage of quantum computing with machine learning algorithms is ushering in a breakthrough in processing power and efficiency. Through optimization of quantum circuits, error mitigation, and simulation acceleration, machine learning algorithms play a key role in pushing the boundaries of quantum computing. Quantum computing has the potential to transform a wide range of industries, including banking and drug development, by using machine learning to unleash hitherto unattainable levels of insight and creativity.
A survey of machine learning applications in quantum computing
In-depth analysis of machine learning applications of quantum computing indicates a vast range of potential uses. Some examples of how machine learning is revolutionizing the field of quantum computing are quantum-enhanced optimization techniques, quantum-assisted data analysis techniques, and quantum machine learning algorithms. These uses highlight how flexible and adaptive machine learning algorithms are when it comes to using quantum resources to effectively address challenging challenges.
Role of Machine Learning in Quantum Algorithm Development
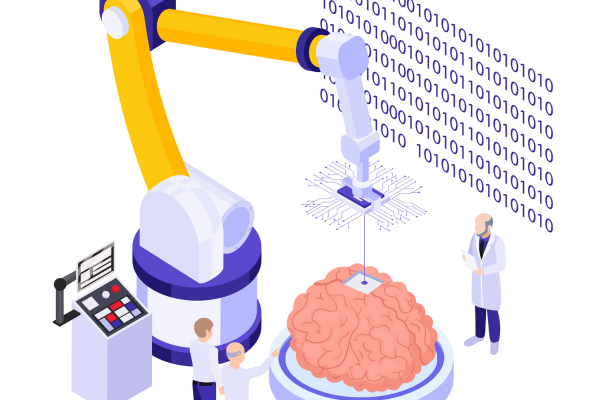
The development of algorithms in the quest to advance quantum computing relies heavily on machine learning. Machine learning algorithms help in finding new quantum algorithms and improving already existing algorithms using data analytics and optimization approaches. This cooperative approach has accelerated the development of quantum algorithms, opening the door to useful applications in machine learning, cryptography, and optimization.
Role of Machine Learning in Quantum Algorithm Design and Optimization
Quantum algorithm construction and optimization requires the use of machine learning techniques. The best quantum algorithms and circuit designs are selected using machine learning techniques that analyze large-scale datasets and simulate quantum systems. Machine learning speeds up the development cycle by automating the algorithm refinement process, guaranteeing that quantum algorithms are optimized for optimal performance and efficiency.
How quantum computing principles are inspiring the next generation of machine learning algorithms?
The building blocks of quantum computing, such as entanglement and superposition, provide a wealth of ideas for the advancement of machine learning algorithms. These ideas are used by quantum-inspired machine learning algorithms to overcome the constraints of traditional computing and provide new methods for pattern recognition, optimization, and decision making. Machine learning algorithms provide new perspectives in computing effectiveness and efficiency by adopting quantum concepts.
Important methods and processes for harnessing quantum computing power
To fully harness the promise of quantum computing, one must be proficient in the key technologies and protocols. Effective use of quantum resources is supported by fundamental ideas such as quantum error correction techniques, quantum algorithm design principles, and quantum hardware optimization strategies. Researchers and practitioners can take advantage of the unmatched processing power of quantum computing to solve real-world problems in a variety of disciplines by incorporating these techniques into useful applications.
Conclusion
In short, computational science is undergoing a paradigm shift as a result of the convergence of machine learning and quantum computing. We can expand the boundaries of creativity and discovery by merging the capabilities of these two fields in a synergistic manner. The combination of machine learning and quantum computing has the potential to fundamentally and profoundly change the technological landscape, from accelerating scientific research to revolutionizing entire fields.
FAQs
By using the specific capabilities of both domains, machine learning algorithms are easily incorporated into quantum computing through methods including quantum machine learning, quantum-assisted data analysis, and quantum-enhanced optimization.
By making it easier to find new algorithms, optimize quantum circuit designs, and automate the refining process, machine learning plays a vital role in the creation of quantum algorithms and advances the field of quantum computing research.
Principles of quantum computing, such as superposition and entanglement, provide new computational paradigms and insights that facilitate creative approaches to problem-solving and decision-making, which in turn inspire the creation of next-generation machine learning algorithms
Pingback: Quantum machine learning algorithms for drug discovery applications
Pingback: Machine learning algorithms for crop yield prediction