Introduction
With increasing awareness about environmental concerns in the society, assessment and forecasting of air quality has become an essential task. Thankfully, technological advances—in particular, the use of machine learning algorithms—have created robust tools to successfully deal with these obstacles.
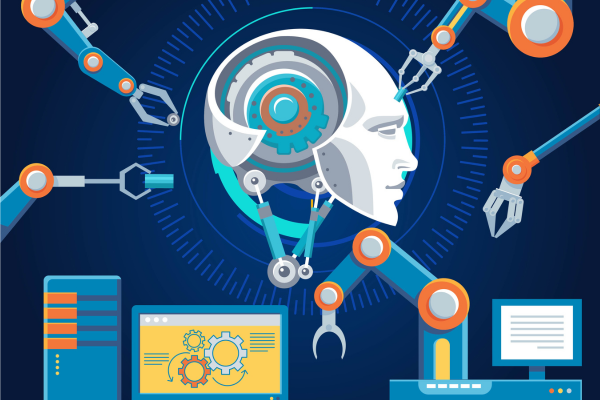
Table of Contents
Machine Learning and Air Quality Modelling
Air quality modeling has been revolutionized thanks to machine learning algorithms, which provide flexible and dynamic solutions. Machine learning algorithms, unlike traditional techniques that rely on predetermined equations, are able to examine large datasets and identify minute patterns, generating more accurate predictions and insights.
Neural networks and decision trees are two examples of machine learning systems that are capable of processing complex correlations between different environmental elements and air pollution levels. These algorithms become more accurate over time by continuously learning from fresh data, making them indispensable tools in the fight against air pollution.
Data-driven approach to air quality forecasting
Machine learning in air quality modeling largely depends on data-driven methods, which is essential for its success. Machine learning algorithms are able to accurately predict future air quality conditions by combining historical data on air quality, meteorological data, and other relevant aspects.
For example, to estimate air pollution levels with previously unheard of accuracy, scientists at the National Center for Atmospheric Research (NCAR) have created machine learning algorithms that examine satellite data and ground-based measurements. With the help of these models, officials can predict pollution patterns days or even weeks in advance, allowing them to take preventive action to protect public health.
Power of algorithms for air quality
It’s amazing how machine learning algorithms can extract hidden meanings from data on air quality. These algorithms can find connections and patterns in large-scale datasets that traditional approaches might miss. This skill enables scientists to understand at a deeper level the complex relationships that exist between pollutants, weather cycles, and human activity.
For example, scientists in Beijing, China analyzed data on air quality and used machine learning algorithms to determine the primary causes of pollution in the city.
Machine Learning in Air Quality
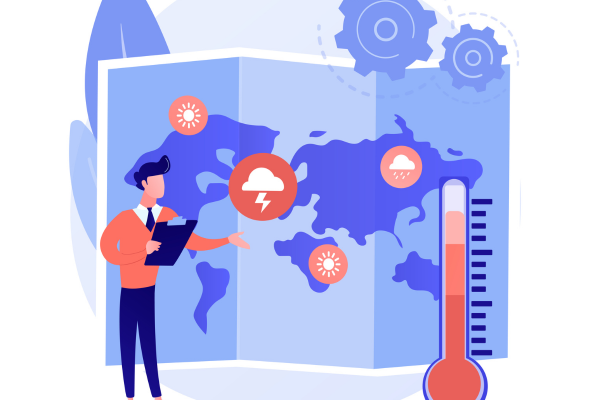
The field has changed as a result of the use of machine learning in air quality management and monitoring. By automating data analysis processes, these algorithms provide the latest information on air quality. For example, machine learning algorithms integrated into air quality monitoring networks enable detection of pollution increases and prompt responses from authorities.
Additionally, sophisticated datasets can be analyzed by machine learning algorithms to pinpoint pollution hotspots and evaluate how well emissions control strategies are working. These algorithms enable policy makers to make informed decisions to protect the environment and public health by providing practical insights.
Machine Learning for Air Quality Forecasting
Forecasting is one of the most important uses of machine learning in air quality modeling. To effectively predict future air quality conditions, machine learning algorithms can evaluate historical data, weather forecasts, and other relevant information. With the help of these estimates, officials can reduce the negative effects of poor air quality on public health by implementing preventive measures such as traffic limits and health advisories.
For example, the European Union’s Copernicus Atmosphere Monitoring Service (CAMS) produces daily air quality forecasts for cities across Europe using machine learning algorithms. These estimates provide useful information to decision makers that enables them to act proactively to reduce pollution levels and protect public health.
Environmental Science with Machine Learning
Because machine learning makes it possible for scientists to analyze large datasets more quickly, it has opened up new possibilities in environmental science. These algorithms can find patterns and trends in environmental data, providing information that can guide scientific studies and policy choices.
For example, machine learning algorithms were employed by academics at Stanford University to detect deforestation patterns in the Amazon forest by analyzing satellite footage. Policy makers can protect this important ecosystem by identifying areas at high risk of destruction and implementing targeted conservation measures.
Air Quality Modeling Techniques
The complexity of real-world conditions is often beyond the capabilities of traditional air quality modeling tools. A more adaptable and flexible method is provided by machine learning, which enables models to evolve and improve over time. These algorithms can analyze a variety of datasets, such as satellite images, ground-based measurements, and atmospheric simulations, to provide accurate predictions of air quality conditions.
For example, researchers at the University of California, Berkeley created a machine learning model called “DeepAir” that combines satellite images with ground-based measurements to predict air quality levels with high accuracy.
Machine Learning for Clean Air
The ultimate goal of using machine learning in air quality modeling is to achieve better air for all. These algorithms enable officials to take proactive steps to reduce pollution levels and protect public health by identifying pollution sources, optimizing emission control systems, and predicting changes in air quality.
For example, machine learning algorithms were used by Indian researchers to detect traffic patterns and areas with increased car emissions. Through the implementation of traffic management strategies, including vehicle limits and congestion charging, authorities were able to reduce pollution levels and enhance air quality in metropolitan areas.
Conclusion
Modeling and control of air quality has been transformed by machine learning algorithms, providing effective tools for protecting the environment and public health. Through the use of sophisticated modeling techniques and data-driven methods, these algorithms provide critical insights into the state of air quality, allowing authorities to take preemptive action to reduce pollution levels. As long as we continue to use machine learning, we can strive towards a future with healthier communities and cleaner air.
FAQs
Predictions made by machine learning for air quality can be quite accurate, especially when combined with sophisticated modeling approaches and trained on large datasets.
Many uses of machine learning include predicting air quality, identifying pollution sources, modeling emissions, and optimizing air quality control technologies.
To enhance air quality in their communities, policymakers can use machine learning insights to make data-driven choices, take focused initiatives, and allocate resources more efficiently.