Introduction
The foundation of OpenAI’s conversational AI model, ChatGPT, is a sophisticated neural network architecture. We will examine in detail in this blog article the development, construction, training, and applications of the neural networks that ChatGPT uses. Gaining an understanding of these aspects will provide a complete understanding of the functioning of this powerful AI model and its implications for Artificial Intelligence going forward.
Table of Contents
GPT’s Evolution between GPT-1 and GPT-4
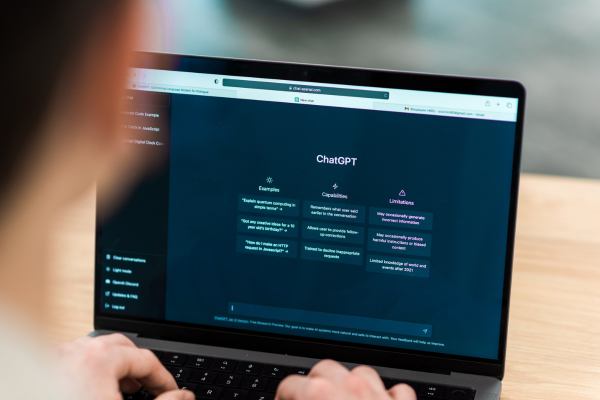
GPT-1, the initial version of the Generative Pre-trained Transformer model, marked the beginning of ChatGPT’s adventures. With a medium-sized neural network trained on a wide variety of online content, GPT-1 laid the foundation. Building on this, GPT-2 used a larger dataset and more parameters to dramatically enhance the model’s capabilities. The subsequent leap, GPT-3, unveiled a model that had 175 billion parameters, one of the largest AI models at the time. Finally, the most recent version, GPT-4, improves the architecture’s ability to produce language that is more similar to a person’s language and accomplish challenging tasks.
Architecture of GPT-4
The Transformer neural network, the foundation of GPT-4’s design, processes and produces text through a process called self-attention. This design is composed of multiple layers of transformers, with feed-forward networks and attention leading at each level. GPT-4 is able to produce coherent and contextually relevant text thanks to the ability for the self-attention mechanism to weigh the relevance of different words in a phrase. The neural network ChatGPT relies on this sophisticated design at its heart to achieve its remarkable performance.
Ethics in training ChatGPT and other neural networks
There are important ethical issues when training neural networks like ChatGPT. The model’s ability to generate biased or harmful information is one of the main concerns. To address this, OpenAI uses several strategies, such as developing rigorous testing procedures and using a variety of training datasets. The environmental impact of training large AI models is also a problem as it requires significant computational resources. Dealing with these ethical dilemmas is imperative to develop and apply AI technology responsibly.
The Power of Transformer Neural Network ChatGPT
In ChatGPT, transformers serve as the neural network core. The transformer model, which made its debut in the “Attention Is All You Need” paper, transformed natural language processing by making it possible to quickly and effectively analyze huge text datasets. The transformers in ChatGPT enable simultaneous processing of input data, which greatly speeds up the training process and improves the model’s text-reading and generation capabilities. Neural networks, such as ChatGPT, rely heavily on this effective processing capability to achieve great performance and accuracy.
Problems and Constraints with Large Neural Networks
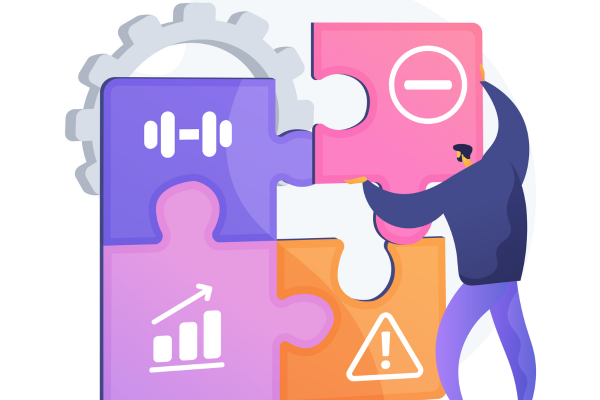
Although GPT-4 and other giant neural networks are incredibly powerful, they also have drawbacks. The computational cost of training and deploying these models is one of the main constraints. Large models can also be vulnerable to overfitting, a condition in which they work well with training data but poorly with new, untainted data. The possibility of producing biased or inappropriate content is another drawback, which requires constant observation and improvement.
Training Large Language Models
Training large language models, such as ChatGPT, involves several steps. The model is first pre-trained using a large corpus of text, which teaches it structure, patterns, and contextual information. After the pre-training phase, the model is fine-tuned using more specific datasets to enhance its performance on certain tasks. The training process takes a lot of time and processing resources to complete, making it a resource-intensive undertaking. To understand what neural networks ChatGPT uses to achieve its capabilities, one must understand this training process.
Fine-tuning
An essential step in ChatGPT development is fine-tuning. In this step the pre-trained model is exposed to more specific datasets, which enables it to become more specialized in particular domains or activities. The model can give more accurate and appropriately relevant answers with the help of fine-tuning. This step is crucial to improve the real-world uses of ChatGPT and ensure that it works well in many industries, from customer service to creative writing.
Contribution of Reinforcement Learning to the Development of ChatGPT
An important part of the development of ChatGPT is reinforcement learning (RL). In reinforcement learning, the model learns to make decisions by gathering inputs from its interactions with the outside world. Over time, these inputs improve the model’s performance. To make the model’s answers more in line with human tastes and expectations, ChatGPT uses reinforcement learning (RL). The neural network ChatGPT uses this iterative learning and development process as a key component to enhance its capabilities.
Applications of ChatGPT
From chatbot development to creative writing assistance: ChatGPT offers a variety of uses, from building chatbots for customer support to helping with creative writing assignments. ChatGPT can handle common customer support queries, leaving human agents free to solve more complex problems. It can help writers in the creative field come up with ideas, create content, and provide comments. These uses highlight the adaptability of ChatGPT’s neural network and its potential to revolutionize many fields.
What is the future of AI for GPT and neural networks?
With the constant improvements being made in model design, training methods, and applications, the future of GPT and neural networks in artificial intelligence is bright. Scholars are constantly investigating ways to enhance the efficacy and efficiency of these models, resolve current bottlenecks, and broaden their scope. Even more advanced models may be developed in the future that can understand and generate even more complex and nuanced language, greatly expanding the capabilities of the neural network ChatGPT.
Conclusion
In summary, understanding the neural networks employed by ChatGPT provides important insights into how this sophisticated AI model functions. With its complex transformer architecture, training procedures, and evolutionary path through multiple GPT versions, ChatGPT is a remarkable achievement in the field of artificial intelligence. Looking ahead, continued research and ethical issues will be crucial in determining the direction of the next wave of AI technology.
- You might be interested in reading these posts as well
- Which are Common Applications of Deep Learning in Artificial Intelligence?
- Which machine learning algorithms uses both labeled and unlabeled data for training?
- What is Deep Learning and its Applications?
- Is deep learning harder than machine learning?
FAQs
The Transformer model is the main neural network architecture used in ChatGPT. It processes and generates text using self-attention techniques.
By adding additional parameters and training data, ChatGPT has progressed from GPT-1 to GPT-4, providing better text generation capabilities and improved performance.
To ensure that ChatGPT is trained ethically, biases in the training data must be addressed, the model must not produce harmful content, and the computing resources required must have an ethical impact on the environment.
By exposing the pre-trained model to more specific datasets, fine-tuning the performance of ChatGPT enables it to specialize in certain activities and deliver more accurate and relevant answers.
ChatGPT can be used to build chatbots for customer service, help with creative writing tasks, and serve various sectors by generating content and automating repetitive queries.